A/B Testing Design Tips to Formulate Valid Marketing Experiments
Author & Editor
Founder & CEO
Published on: Sep 14, 2023 Updated on: May 21, 2024
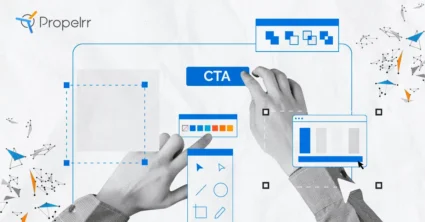
Table of Contents
A/B testing design is a crucial part of every digital marketer’s success.
Whether you use it for pay-per-click (PPC) advertising, user experience (UX) design, or any other digital execution, this pillar of marketing experimentation will help you identify areas of poor performance, create strategies for improvement, and optimize results for your every campaign.
To create effective A/B testing for UX design, content creation, and other forms of digital marketing, you need to know how to create test variants that are not only valid, but reliable as well. With the help of this data-driven guide by Propelrr, you can make sound business decisions that’ll help drive digital marketing wins for your brand’s benefit today.
Ready to take a deep dive into split testing and variant design? Then check out this guide to learn more right now.
Understanding A/B testing experiment design
How can you tell if a PPC ad is working or not? Are there ways for you to optimize one for the benefit of your future campaigns? The answers to these questions are simple: you can tell if your ad is working or not and optimize it for future campaigns, with the help of data-driven A/B testing.
Also known as split testing, this form of experimentation is done to test two or more variations of a social media ad, landing page, copy, or other aspect of online advertising. It helps marketers see which variation of an execution works best for the goals of a business, thus allowing you to optimize more campaigns in the long run.
A basic split test pits an original version “A” against a variant version “B,” hence the “A/B” name. A multivariate test, on the other hand, compares a higher number of variables against one another. A multi-page experiment tests consistent variations across multiple pages, thus allowing for even more optimization across a higher number of landing pages.
Testing helps marketers in a myriad of ways. You can use it to make crucial campaign decisions, areduce low-performing ads losses, and even bolster landing page performance. You can also use this form of experimentation to optimize almost any execution. From social media formats to ad word optimization, split tests can uncover tons of ways to improve paid marketing efforts today.
How to design A/B testing that is valid and reliable
Given the value of testing for your digital marketing campaigns, it’s only natural for you to want to run accurate A/B analyses that return helpful results. To do that, you need to formulate valid and reliable test variants that are based on data in order to gain insights that bolster the performance of your executions rather than bring them down.
To create a test that returns data-driven results for your brand, all you need to do is follow these five steps:
1. Research your “why”.
Before you start any A/B testing run, you need to do your research to understand why you’re experimenting in the first place. What campaign or aspect of your marketing are you analyzing, and why do you want to improve upon that for your business?
Without this pre-experiment research, you won’t have a clear idea of what digital marketing objectives you want to achieve overall. Are you trying to find a call to action (CTA) that best garners conversions for your sales landing page? Are you comparing the efficacy of your marketing email headlines?
Study your chosen execution first, and conduct research to see if there are things you can improve before you run any tests. Find out which metrics you need to measure the marked improvement of your campaign, like click-through rate, conversions, engagement, and more.
2. Formulate a clear hypothesis.
The next thing you’ll want to do is to formulate a clear and specific hypothesis for your analysis. This hypothesis should state the problem you are trying to solve, the metric you’re trying to improve, and the change you’re trying to see in your campaign. A basic example of a hypothesis might look like this:
Changing the color of a CTA button from blue to red will increase the number of clicks by 10% in two weeks.
Of course, this hypothesis will only be useful to you if you root it in the research you conducted in the previous step. Don’t make the mistake of creating a hypothesis out of nowhere; make sure to research and study your past data, campaign objectives, and metrics, in order to formulate an effective hypothesis for your experiment.
3. Create variations.
This step is the most important in this guide. In order to create valid and reliable variants for this comparison, you need to define your “control” or variable A, and “challenger” or variable B, based on the well-researched hypothesis you set up in the previous step.
Some examples of highly specific variables to create variants for include:
- Headlines
- Copy
- CTAs
- Images
- Backgrounds
- Colors
- Sizes
- Placement
- Audiences
- Demographics
These are just a few of the myriad of variables that you can make test variants for today.
If it’s your first time conducting this type of marketing experiment, then you should only compare one variable at a time. If you compare wildly different variables against one another, or create variants with too many different variables, then you’ll have a harder time monitoring data and pinpointing accurate findings for your campaign.
By comparing just one variable against another in your variants, you’ll return the most accurate results for your experiment - thus ensuring reliable improvements for your landing page, UX design, social media ad, or email body in the long run.
4. Run the test.
Once you have your research, hypothesis, and variants in place, you can finally run the test campaign for your digital marketing execution. Again, remember to keep all other variables untouched among your variants so that you can actually gain accurate results from your experiment. Otherwise, you’ll get vague and unhelpful outcomes, thus wasting your precious time and resources for the campaign.
5. Analyze results and deploy changes.
The last thing you’ll have to do when running your A/B experiment is to analyze your results and deploy the respective change. How did the control and challenger variants fare in hitting your set metric? Did you notice any significant differences or anomalies throughout the duration of your experiment? How confident are you in the accuracy of your results?
With the right variants for your analysis, you should be able to deploy a new and improved way to make your chosen execution more effective. Of course, there’s always more room for optimization - you can optimize other elements on your landing page, study another aspect of your UX, and even run another variant comparison based on the findings of your first test.
With this step-by-step guide to A/B testing and experimentation in mind, you can now discover more refined ways to craft effective variants for your optimization journey today. Keep on reading to discover expert tips to improve your own test variants for a future split test.
Things to prepare for A/B testing system design
In addition to conducting prior research for your upcoming comparison, you’ll need to prepare a few other things in advance first. To create an A/B testing system design that produces valid and reliable results from your variants, you need to:
- Define your success metrics. Success metrics are quantitative indicators you use to evaluate the performance of your variants, in order to determine the winning variation. These metrics should be reflective of your overall business goals, and can include examples like click-through rate, conversions, and more.
- Segment your target audiences. To effectively run comparisons on variants, you should segment your test’s target audiences first. Segmentation refers to how you divide target audiences into meaningful subgroups, based on characteristics or behaviors relevant to your experiment. These might include segments based on age, gender, location, and more.
- Enact randomization to reduce bias. Before unleashing your analysis on target audiences, enact randomization ahead of time to reduce bias in your overall results. Randomization is when you assign audiences to each variant randomly and evenly; this reduces selection bias and ensures fair comparability between groups.
Given all these system design preparations you need to do beforehand, you’ll need to employ the help of an A/B testing tool or platform to automate the process, save on money and effort, and optimize your experiment design efficiently today.
Tips for crafting effective variants
Once you’ve prepared a fair and reliable system design, you can then craft variants with which to run an efficient A/B testing methodology. To do this, make sure to:
- Design variants that align with your hypothesis. By rooting your variant in the research conducted and the hypothesis set for your analysis, you’ll be able to answer your problem question more easily and ensure testing results that reflect changes in your campaign more clearly too.
- Implement significant and measurable changes from your variant. Once you finish running the test and picking the better variant, implement the changes from said variant to see if it really improves your campaign, UX, or marketing execution. Then, make sure to measure these changes for future optimization and experimentation.
- Avoid potential pitfalls in variant creation. Don’t test multiple variables at once, or else you’ll muddy the testing pool and garner vague results. Remember to determine your target audience beforehand so that you can run your experiment on the appropriate user segment. Lastly, identify how long you plan on running the analysis to achieve statistical test significance.
By following these tips to craft your variants, you’ll garner clearer results that communicate the exact changes you need to make to optimize a digital marketing execution for your business’ success in the long run.
Test reliability and reproducibility
Want to ensure the reliability and reproducibility of your research design and testing methodology across all campaigns? Here are some tips to take into consideration today:
- Understand the types of test validity. Internal validity refers to how your methodology is able to isolate changes from a variant (versus changes from other factors), thus making your results more valid. External validity refers to how your research design can be made general enough to apply to external findings from other situations, like different devices, browsers, or audience segments. By understanding these types, you can track the validity of your results and reliably reproduce your design with other variables and variants.
- Identify threats to your test’s validity. Identify all potential threats to the validity of your research design, like improper audience segmentation, test duration, or sample size. Then, make sure to remove these threats from your methodology to improve its overall reliability and reproducibility.
- Minimize confounding variables. Confounding variables are variables that turn up in the middle of an A/B testing run, thus posing a threat to the validity of your results. Examples include updates to search engine algorithms, website or server outages, and other sudden changes. To minimize the risk of confounding variables, make sure to track all potential threats and to time your run wisely to avoid running into unexpected changes to your test.
Consistency is key when it comes to A/B analysis. Make your research design reliable and reproducible by ensuring consistency throughout the duration of your initial split analysis run.
How to analyze A/B results accurately
To accurately analyze the results of your A/B experiment, always go back to your original hypothesis. Did the end result meet the expectations of your problem statement, or did it show the opposite? What does it mean if you returned results that were opposite to your expectations?
Once you go back to your original hypothesis, make sure to visualize the data you collected from the experiment’s run. This will help you analyze your findings more clearly, thus helping you mine more insights from other factors like your audience segments, duration, or sample size.
Should you find it difficult to execute this part of the A/B analysis, reach out to experts in your field to get accurate analytics for your business’ needs today.
Key takeaways
Design your split testing like a pro when you know how to craft effective variants for comparison today. Here are some final reminders to take with you as you begin your research into A/B analysis for digital marketing success:
- Root your strategies in data. Whether you’re formulating a hypothesis or creating a variant for comparison, make sure to root your choices in data from previous marketing campaigns to ensure the accuracy and relevance of the results you’ll gain.
- Take your time in crafting the experiment. You can’t rush into a split test and expect to return reliable results right off the bat. Take your time in crafting the analysis to ensure the reliability of your findings and the reproducibility of your research design.
- When in doubt, reach out. Not too confident in your A/B testing skills just yet? Don’t be afraid to avail expert digital marketing services from Propelrr for skilled analyses and marketing experimentation today.
If you have any other questions, send us a message via our Facebook, X, and LinkedIn accounts. Let’s chat!
Subscribe to the Propelrr newsletter as well, if you find this article and our other content helpful to your needs.h