A/B Testing Significance: Guide to Validate Marketing Experimentations
Author & Editor
Founder & CEO
Published on: Oct 12, 2023 Updated on: May 16, 2024
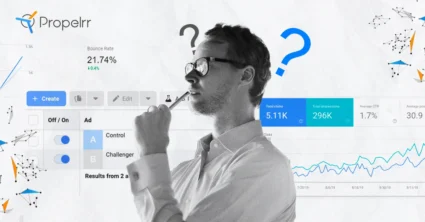
A/B testing’s significance cannot be underestimated in the data-driven world of expert digital marketing. It is a crucial process that must be conducted properly, in order to garner the best possible results for any brand online.
Of course, there’s more to A/B analysis than meets the eye. As a digital marketer, you need to understand how to correctly interpret an experiment’s findings - else you risk running costly campaigns for pay-per-click (PPC) ads or social media based on faulty data and information.
To achieve digital marketing success for your business, it is imperative that you know how to validate your split test findings properly. To do that, you need to know how to test for significance - and you can do that with the help of this guide to statistical significance from digital marketing services today.
Learn how to interpret and validate the significance of your A/B testing results the right way for all your digital marketing needs. Check out this guide to discover fundamental concepts, address misconceptions, and apply techniques for PPC and other executions in your digital marketing strategy right now.
Statistical significance: Its significant value to A/B testing
Before you go deep into the tips and techniques for interpreting and validating findings, you need to understand the importance of statistical significance to your split analysis needs first.
In the context of running A/B tests, statistical significance refers to the likelihood of your findings being valid, and not a result of error or random chance. You’re comparing a control and a variant in a split analysis, to see if the differences between the two are significant enough to warrant action. If, for example, your variant is statistically proven to perform better than your control, you can then apply the former to your marketing campaign in order to optimize it for business wins.
This data-driven measure can help you validate your split analysis findings, thus ensuring the optimization of your subsequent campaigns. It is therefore an important part of your marketing experimentation process because it ensures the validity of your findings for surefire success.
The significant value of a test’s hypothesis can be measured in many ways. One of the best ways you can test the statistical significance of a hypothesis is through its p-value. Simply put, the p-value refers to the likelihood of your alternative hypothesis being correct; this means that your variant is statistically proven to be better than your control in the overall A/B analysis.
Think you’ve got the basics of this measure and the relevance of the p-value down? Then let’s get into some tips and practices for formulating hypotheses for your experiment next.
How to formulate hypotheses for your A/B tests
To be able to experiment with marketing content, you need to know how to properly formulate a hypothesis first. Here are some tips, types, and common things to avoid when you formulate a hypothesis for your testing needs:
Choosing the right hypothesis metrics
To be able to design a hypothesis, you first have to establish your metrics for measuring your experiment. In choosing metrics, make sure to align them with your brand’s overall objectives and goals. This is so that you can measure and garner results that positively impact your business needs. Some common metrics for A/B analyses include:
- Conversion rate - One of the most common metrics for split experimentation, your conversion rate refers to the number of users who complete a desired action on your website, ad, or other marketing campaign.
- Click-through rate (CTR) -. Your CTR, on the other hand, refers to the number of users who click through to your desired landing page through your ad or product listing.
- Bounce rate - Lastly, your bounce rate refers to the number of people who quickly leave or “bounce” from your website, page, or campaign without taking additional actions.
There are many other types of metrics that you can choose from when you formulate your hypothesis. Of course, your choice of metric will depend on what you’re trying to measure and achieve for your brand’s digital marketing success.
Types of hypotheses in A/B testing
As you formulate your assumptions and conduct marketing experiments, you’ll need to differentiate the various types of hypotheses available to properly interpret and validate your subsequent results. The types of hypotheses for split experimentation include:
- Null hypothesis - The null hypothesis type states that there is no statistical relationship between your control and your variant; simply put, this implies that no change occurred based on the results of your comparison.
- Alternative hypothesis - The alternative hypothesis type, on the other hand, states that there is a statistically significant difference between your control and variant. This implies that change did occur, based on the results of your split analysis
- One-tailed hypothesis - Also known as a directional test, a one-tailed hypothesis accounts for one scenario and explores if your variant is better or worse than your control. Two examples of such a hypothesis might state that there is an increase or a decrease in your website CTR, due to the use of a specific Google ad word in your website’s headline.
- Two-tailed hypothesis - This type, on the other hand, accounts for multiple scenarios and explores if there is a difference between your variant and your control at all. Three examples of such an assumption might state that there is either an increase, decrease, or no change, in the conversion rate of your landing page due to a change in color for your call to action (CTA) button.
You’ll see these terms pop up a lot when you test statistic value, but simply put, these hypotheses help you establish an assumption you’d like to test for the sake of improving a marketing campaign, like a Facebook ad or some website content.
Crafting effective hypotheses
If you’re on the lookout for tips to craft an effective hypothesis, then you’ve reached the right section. Here are some helpful techniques to craft a testable assumption that’ll help you optimize any digital marketing campaign:
- Be SMART. That is, make sure to set specific, measurable, attainable, relevant, and time-bound objectives (or SMART objectives) for the experiment.
- Avoid ambiguous assumptions. Putting an emphasis on specificity, avoid writing ambiguous assumptions and make sure your hypothesis states a specific problem that’s solvable with your split analysis.
- Test one thing at a time. You’ll also want to avoid a hypothesis that asks you to test multiple variables in one go; such an overly complex experiment will return confusing, inconclusive, and inaccurate results.
Want to understand these tips in action? Some real-world examples of marketing campaign hypotheses from real-life businesses include:
- MANGO - Wanting to test the effectiveness of Facebook’s Advantage+ shopping ads versus its older, more fragmented Facebook catalog ads, MANGO compared performances and saw a 58% higher reach for their Advantage+ shopping campaigns, versus their usual Facebook campaigns.
- Tropicana - This popular juice brand wanted to see if adding Instagram Reels to its usual video ad placements - Facebook Feed and Stories, and Instagram Feed, Stories, and Explore - would improve brand awareness. By running an A/B analysis to compare ad placements, Tropicana saw a 4.1-point lift in ad recall when running video ads in their old placements, plus Reels.
- Bostani Chocolatier - Hoping to track an increase in sales, Bostani Chocolatier ran a split test to compare the before and after results of a TikTok In-Feed Video Ad campaign. The test showed a 15% increase in website traffic and a 20% increase in sales, all because of their in-feed video ad.
By crafting specific and attainable hypotheses for your marketing experimentation, you can garner clear and unambiguous results for your campaign’s overall improvement. Of course, you have to test the validity of your findings to ensure the security of this optimization - which is why we’ll get into misconceptions and challenges you might encounter when you test statistical value next.
Common misconceptions when you test statistic value
As you learn how to validate the results you garner from split analyses, you also need to keep an eye out for certain misinterpretations of your findings’ measures and data. Here are some common misconceptions to avoid when you test the value of your statistical significance.
The lower the p-value, the higher the significance
While it’s generally accepted that a lower p-value means a higher likelihood of your alternative hypothesis being true, you have to remember that this assumes your null hypothesis is also correct. If your null hypothesis is incorrect or based on faulty assumptions, then your experiment and its results won’t be sound.
To confirm the validity of your comparison, do repeated runs to validate the statistically significant relationship between your null and alternative hypotheses.
Statistical significance equates to practical significance
Statistical significance doesn’t automatically imply practical or real-world relevance, because no statistical test can be accurate or large enough to measure results for your entire target population. This is where your expertise as a digital marketer comes into play - to observe your audience and see if your statistical results match real-life user behaviors.
Statistical significance ensures replicability
Replicability refers to the ability of a test to be repeated using the same methods, with new data, but with the same results. This lets marketers believe that the results of the original study remain totally reliable, even with new data.
However, it can be difficult to replicate any study, since outside factors can easily affect the results of any experiment. So it’s better to debunk the idea that the significant measure from one study can be wholly and totally applicable to another similar and replicated study.
Significance levels are all the same
Significance level refers to your level of confidence in rejecting the null hypothesis as true in your study. Not all levels are the same; they can take on values like 0.1, 0.05, and 0.01, with a lower decimal level signifying a lower chance of error (and a greater amount of confidence in your findings).
Statistical significance equates to causation
Just as statistical significance doesn’t equate to practical relevance, it also doesn’t automatically imply causation. Just because a hypothesis and a result hold a strong correlation to one another, does not mean that one definitely caused the other. You still need to run multiple tests and consider external factors to prove causation.
Statistical significance is immune to bias
Just because a result has statistical significance, doesn’t mean it is immune to bias. Whether deliberate or not, biases can occur when your opinions or beliefs cause inaccurate or incomplete interpretations of results. To mitigate bias, make sure to use careful research design and random sampling procedures for your audience.
Statistical significance is forever
Research is ever-evolving, especially in digital marketing. The only permanent thing among your users is change; therefore, it is impossible for the results of a statistically significant study to be permanent or remain the same forever.
This is why it is important to constantly test and experiment with your campaigns - so that you can continue to improve them even as your audiences change over time.
Beyond the p-value calculator: Navigating common challenges
As you explore more and more forms of experimentation for your brand’s marketing campaigns, you’ll find yourself navigating challenges throughout your journey of validating findings and results. Here are some common pitfalls you’ll face, and how you can handle them properly:
- Small sample sizes - Too small a sample size can decrease your statistical power. Make sure to utilize tools that calculate the right sample size so that you run an effective test.
- Inconsistent results across repeated runs - If you're running into inconsistent findings or failed A/B tests across multiple repeated runs, consider reviewing your hypothesis to see if it's too vague or too specific.
- Results that change over time - It's inevitable that findings change over time, due to the ever-evolving nature of your target market's wants and needs. Conduct experiments constantly to track changes and adjust your strategy accordingly.
- External factors and influences - Mitigate the influence of external factors by conducting runs during stable periods and doing random sampling to avoid bias.
All these pitfalls and tips for handling them should help to make your new statistical journey a lot easier to fulfill. Soon, you'll be able to confidently validate collected data for your brand and develop more sound strategies for future digital marketing executions. So let’s continue on this road to confident test interpretation for better successes for your business today.
The road to confident test interpretation: demystifying the process
To be able to build confidence in your collected data and findings, you'll want cleanly executed runs and automated analyses to validate your results. To optimize this process and ensure long-term significance, you can make use of tools like statistical significance calculators and online dashboards to streamline your work.
These tools and tips can help to make your life a lot easier, thus demystifying the overall process of statistical analysis. Once you harness the powers of properly interpreted data, you can easily pursue informed decision-making in your marketing and drive better digital wins with the help of these data points and interpreted findings today.
Key takeaways
Validate your findings with the help of split tests today. Here are some final tips for you to remember as you run your experiments for your brand’s digital marketing campaigns this year:
- Use statistical significance to bolster the validity of your findings. By using this form of data analysis, you can ensure the accuracy of your results for more secure optimization.
- Balance interpretations with real-life audience contexts. Of course, make sure to consider the human aspect of your analysis for a more holistic understanding of your audience and its needs.
- Make data-driven decisions with expert backing. Harness the power of data-backed digital marketing strategy with the analytics experts at Propelrr today.
If you have any other questions, send us a message via our Facebook, X, and LinkedIn accounts. Let’s chat!
Subscribe to the Propelrr newsletter as well, if you find this article and our other content helpful to your needs.