A/B Testing Plan Tips to Avoid Common Experimentation Pitfalls
Author & Editor
Content Specialist
Published on: Oct 24, 2023 Updated on: May 22, 2024
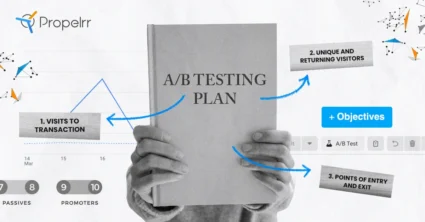
Table of Contents
A/B testing is a pillar of marketing experimentation, and knowing how to make a good A/B testing plan is a critical skill for any business and their expert digital marketing agency.
But as important as it is to know how to build strong split analyses, it’s also just as easy to make mistakes throughout the building process. Without the right services or guidance, you run the risk of making simple testing mistakes that can derail your experiment and return unreliable results for your brand.
Whether you’re running assessments to improve your content, pay-per-click (PPC) advertising, user experience (UX) design, or other marketing executions, you’ll need help to navigate through the common pitfalls of A/B analysis. With the right guidance, you can develop a robust testing strategy that’ll produce reliable insights consistently for your brand today.
Discover common mistakes marketers often make in their experimentation journey, and understand ways to avoid them for your own strategies with the help of this comprehensive guide. Learn more about best tips and practices for building tests for your content, PPC, or UX design when you take a look at everything this Propelrr guide has to offer you right now.
Build an A/B testing plan with a strong foundation
When you build out a well-executed A/B testing plan, you make it possible to collect data and information that contribute to a stronger strategy foundation. By conducting such experiments, you can improve user engagement, content, conversion rates, sales, and more - thus leading to bigger, better digital marketing wins for your brand overall.
This is why it is important to build out split experiments with strong foundations. To do so, you need to define clear objectives, choose the right test variables, and utilize effective testing tools.
- Defining clear objectives. To define clear objectives for your marketing experiments, you need to set measurable goals that align with your overall business objectives as well. This can be done by identifying specific key performance indicators (KPIs) such as clickthrough rates, conversion rates, revenue, and more, to objectively measure your ability to hit goals.
- Choosing appropriate variables. To choose appropriate variables, on the other hand, you need to select the right elements by identifying your experiment’s hypothesis. If you’re testing a website, for example, then you need to state the specific problem you’d like to solve on it - like the effectiveness of a call-to-action (CTA) button, or the visual hierarchy of your content. This hypothesis will allow you to isolate elements and variables that you can analyze using a split experiment today.
- Utilizing testing tools. If you want to bolster the foundations of your experiment, then you can also utilize A/B testing tools for convenience and consistent automation. But overall, you can make a strong foundation for your test process with a clear objective and an appropriate set of variables as well.
Given these specific tips to build out a split analysis with a strong foundation, you might be wondering what it’s like to build one with a weak foundation instead. Continue on to the next section to learn more about the most common pitfalls marketers face when running an A/B analysis right now.
Specific A/B testing plan pitfalls
Discover the mistakes to avoid when running experiments for your brand today. Here’s a list of the 12 most common A/B testing pitfalls for you to circumvent massive mishaps in your experimentation journey:
1. Undefined objectives
As mentioned in the previous section, it’s important to define objectives clearly to build a strong test foundation. If you conduct any type of analysis without a clear objective, you’ll likely return ambiguous results, meaning you’ll have wasted time and resources on a process with minimal to no returns on investment.
The solution? Make sure to clearly define the objectives of your experiment. Think back to your overall business goals - are you trying to increase clickthrough rates or conversion rates? Are you aiming to enhance your user experience? By defining these objectives, you can guide your research design to have a clear path, from start to finish.
2. Lack of hypotheses
One of the most important steps in experimentation is to formulate a hypothesis. Without one, you won’t know what problem you’re trying to solve, thus making it difficult to interpret end results for your business optimization.
Make sure to formulate a hypothesis that attempts to predict the outcome of your test. With this, you can determine the success (or failure) of your assumptions, thus providing you insight into what did and did not work throughout your analysis process.
3. Small sample sizes
Size matters, especially when it comes to your evaluation’s sample size. If you start your analysis with an inadequate number of users or participants, then you may garner statistically insignificant results that can end up being misleading in the analysis stage of your experiment.
To solve this problem, remember to calculate the sample size required to achieve meaningful statistical significance with your assessment. The larger the sample size, the better. Just make sure that you have the time and resources necessary to conduct such an assessment on the sample size of your choice.
4. Biased samples
Not only do you need to ensure that your sample size is right, but you also need to ensure that your sample group is appropriately representative of your target population. If your samples aren’t representative of your target audience, then your results may not actually apply to the needs and contexts of your market.
To address this, you can randomize the selection of participants from your target audience, to ensure that the results per experimental variant are unbiased. This is an important pitfall to avoid, as it can drastically affect the results that are meant to represent your online audiences.
5. Comparing too many variables
Just as you need to be specific about your objectives, you also need to be specific about your chosen variables for comparison.
When you compare too many variables at once, you make it harder to pinpoint the exact cause of your results, thus making analysis more difficult. To fix this, focus on testing one variable at a time in order to isolate the impact of each change accurately. This will provide you with clearer insights from specific changes to your experimental variants.
6. Inadequate test duration
Duration is also an important factor when it comes to the success of your assessments. If you end your analysis prematurely, you won’t garner enough information to formulate a sound conclusion. You may even garner results that are inaccurate or inconsistent, due to variations caused by day-of-week or time-of-day effects.
To avoid this common A/B testing mistake, make sure to run tests long enough to garner sound results. You can use statistical methods to determine the right amount of time for an analysis, or you can use automated tools to determine when you’ve collected enough data for reliable results.
7. Ignoring segmentation
This pitfall relates back to the previously mentioned points on your chosen sample sizes. Even if you select a large enough sample size that is unbiased and reliable, if you fail to analyze your results based on notable audience segments then you may miss out on valuable insights from your audiences overall.
Different user groups react differently to things. So remember to break down the information you garner by relevant segments, like demographics, psychographics, and more, to uncover notable variations in their responses to the experiment.
8. Crafting variations with little change
Another common mistake testers make is to craft variations with too few changes. Even if you run a comparison and garner statistically significant results, if your variation has too few changes then you won’t actually garner any practical outcomes for your brand.
The solution? Consider the real-life impact of the changes you make in your variations. While it’s important to be guided by data and statistical significance, it’s also important to interpret these results alongside the real-world impact of your experimental variants.
9. Ignoring external factors
Like what was mentioned in the previous pitfall, it is very important to be guided by real-world impacts as you run your comparative assessments. If you ignore external and real-life factors such as holidays, economic conditions, and sudden trends, then you also run the risk of inaccurate outcomes for all your analyses as well.
To avoid this common mistake and address the way external factors can influence outcomes, remember to keep track of such events in the real world. Then, make sure to account for these factors during the analysis stage of your evaluation, to ensure that your final findings are holistic and accurate.
10. Confirmation bias
Confirmation bias is the pitfall of interpreting findings based on your own biases, beliefs, and preconceived notions. If you interpret your findings in this manner, then you might accidentally misinterpret the data you collected from your A/B analyses.
To mitigate the risks of this pitfall, make sure to approach your A/B comparisons with an open mind. Do your best to Accept results, whether they support or refute your hypothesis, and use them to refine your strategies
11. Not learning from your mistakes
While this guide is dedicated to avoiding the pitfalls that lead to failed tests, it is inevitable that you may make a mistake or two throughout your long and arduous experimentation journey. Making mistakes is okay - as long as you learn from them and improve upon them in future test iterations.
Every comparison consumes time and resources. So remember to take note of the pitfalls you do end up making, and do your best to avoid them next time around. This will allow you to constantly improve your research design, thus making every future version better than before.
12. Only using A/B testing
Last but not the least is one of the most common pitfalls to avoid throughout your marketing experimentation - only utilizing A/B testing for your brand.
While this form of analysis is crucial to the success of your business optimization, it is only one tool in your massive arsenal of marketing experimentation. This incredibly iterative process can be helpful in some situations, and a hindrance in others. So make sure to expand your toolkit to include techniques like multivariate tests, feedback collection, usability testing, and more, to ensure the creation of the best possible marketing campaigns for your brand today.
Designing a business marketing plan using A/B testing
As important as it is to know what pitfalls to avoid, it’s also equally important to know best tips and practices for designing your business marketing plan using A/B testing. Here are some followup techniques to ensure you’re running an effective analysis for your brand:
- Select appropriate metrics. To identify the right metrics for your business, recall the KPIs you wanted to measure throughout your test. Once you establish these KPIs, you’ll know what metrics to watch out for, in order to achieve objectives for your business.
- Create solid control and variant groups. By specifying these groups accurately, you avoid contamination between the two and ensure more defined analysis results.
- Implement randomization. The random assignment of users to groups can ensure the equal and unbiased distribution of variables. This promises more accuracy out of your results, contributing to a better marketing strategy overall.
- Manage external factors. Identify these external influences, such as acts of god, sudden industry trends, and global crises, so that you can create strategies to minimize their impact on your A/B analysis.
Ethical considerations for your A/B testing plan template
It’s also crucial that you take into consideration the ethics of your split analysis. Are you collecting user data with the knowledge of your target audience? Are you running tests in an inclusive and unbiased manner? Are you being transparent in your testing practice?
By practicing ethics in your evaluation process, you build and maintain a sense of trust between your brand and its audiences. You also mitigate potential biases and risks, thus ensuring the safety and longevity of your business as well.
How to create an A/B testing plan for pages on your website in a meaningful way
At the end of the day, what matters most is that you create assessment plans for your website or platform in a meaningful and well-thought out way. By following the advice detailed in this guide, you’ll be able to craft tests that are effective, meaningful, and impactful - thus driving the success of your brand and its marketing campaigns.
Make experiments of importance with the help of this guide, and the advice of the digital marketing experts at Propelrr today.
Key takeaways
Build out a strong A/B analysis for your marketing campaigns today. Here are a few final takeaways to bring with you as you experiment with your digital for the betterment of your business:
- Be specific and intentional. From choosing test objectives to selecting the right variables, remember to be specific and intentional with the way you run your experiments. This will ensure the clearest possible findings for the purposes of your analysis and interpretation.
- Learn from your mistakes. While it’s important to avoid common pitfalls, don’t let mistakes stop you from implementing the best possible versions of your campaigns for your brand.
- Keep learning. Connect with the experts at Propelrr today to constantly receive feedback and updates about best testing practices for your business too.
If you have any other questions, send us a message via our Facebook, X, and LinkedIn accounts. Let’s chat!
Subscribe to the Propelrr newsletter as well, if you find this article and our other content helpful to your needs.